PRODUCT OVERVIEW
Activate In-Depth
Analytics.
With Kubit, capture full customer journey analytics directly from your existing data warehouse. No ETL or data ingestion.
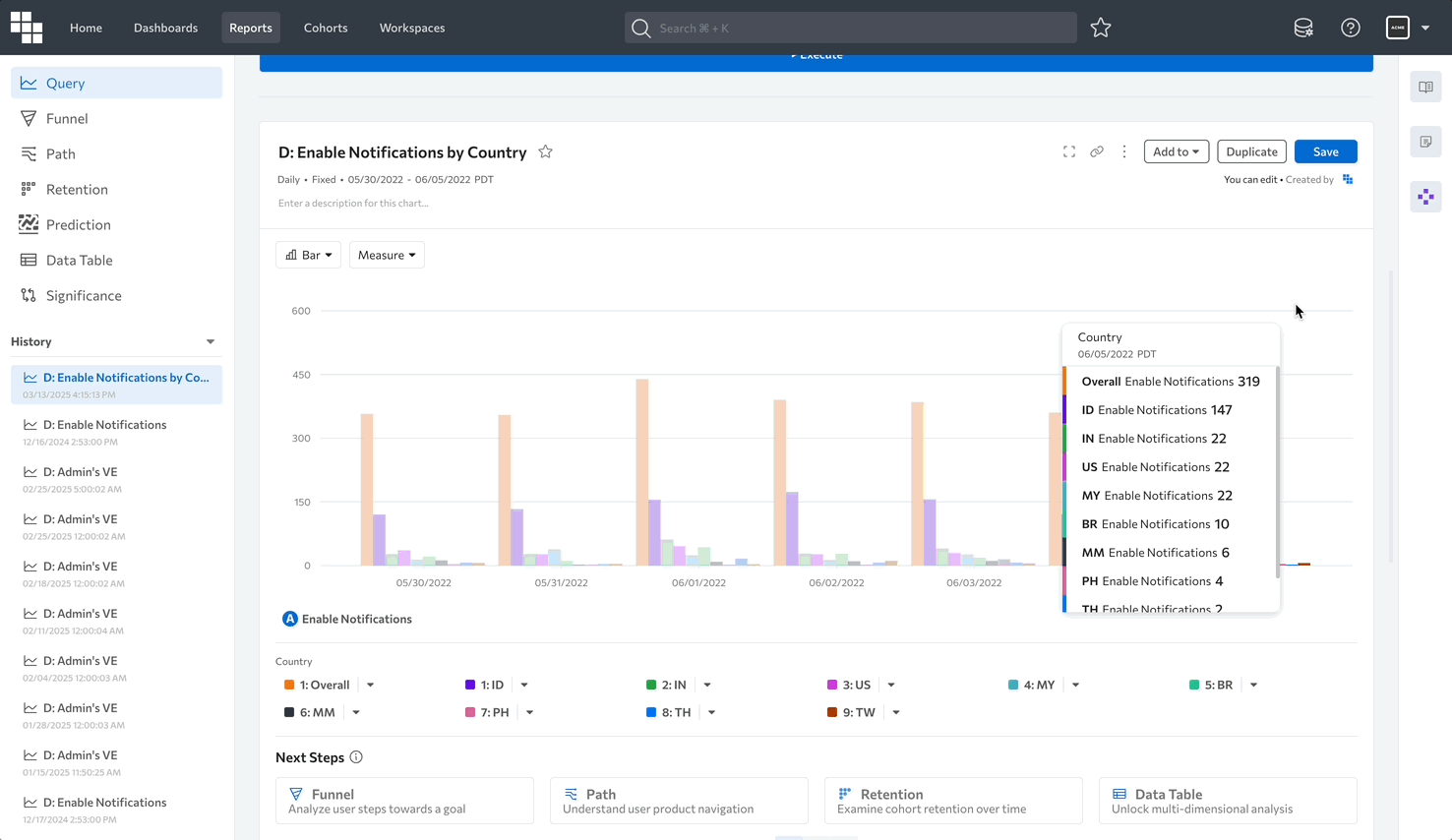
Streamline Data Workflows
Self-Service Data Modeling
Kubit's self-service data modeler allows you to make Schema changes, create Dimension Tables, add Custom Join Conditions, and more—all without ETL or data duplication.
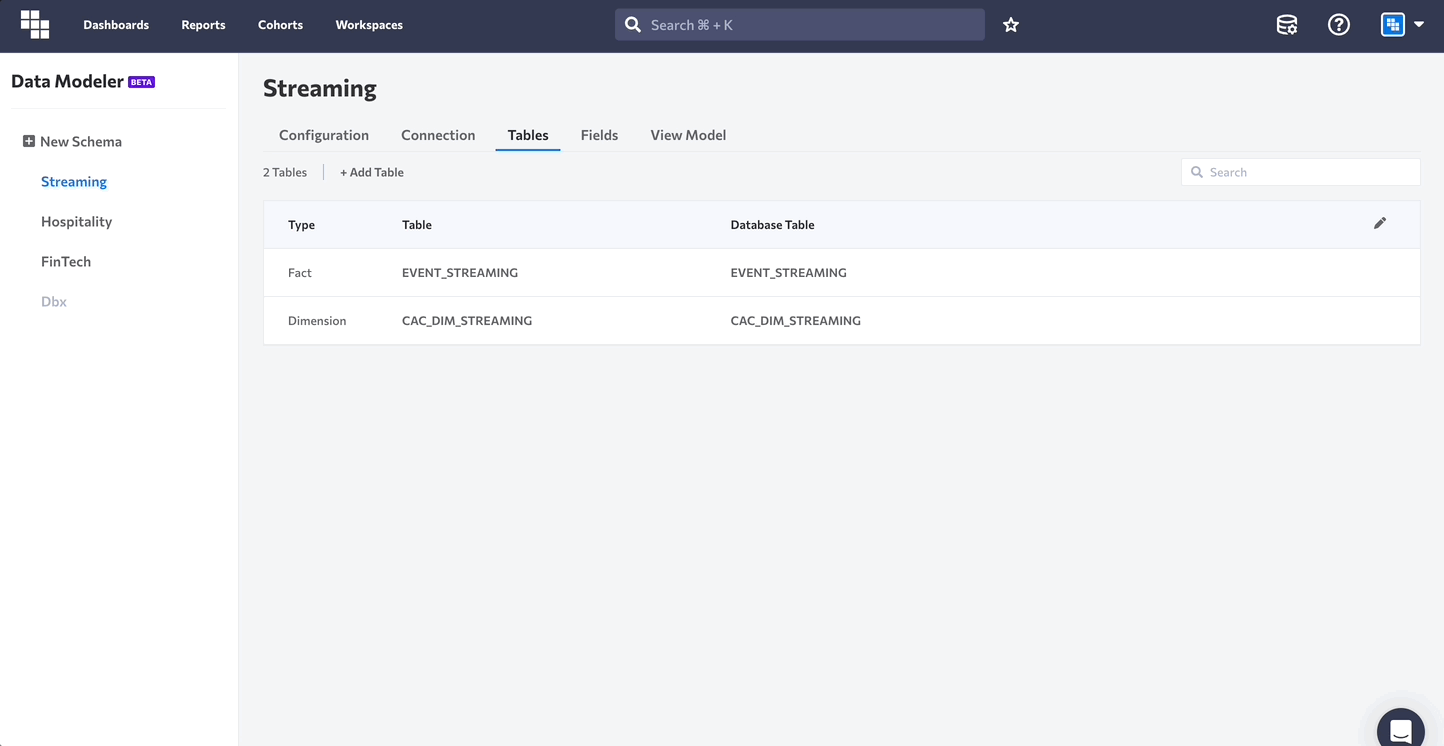
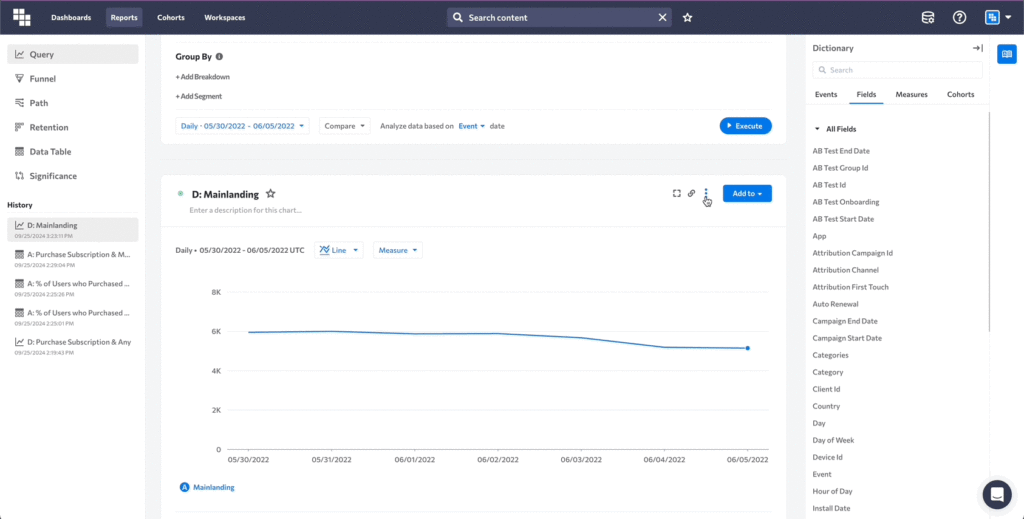
View SQL Behind Reports
Unlike other analytics platforms, you can generate the SQL behind any report with Kubit. This allows you to have full confidence in the integrity of your data—keeping your existing warehouse the single source of truth.
Full Product & CX Insights
From acquisition to retention, Kubit delivers complete insights into every step of the customer journey. Get answers to questions that enable you to improve the product experience and increase your customer base.
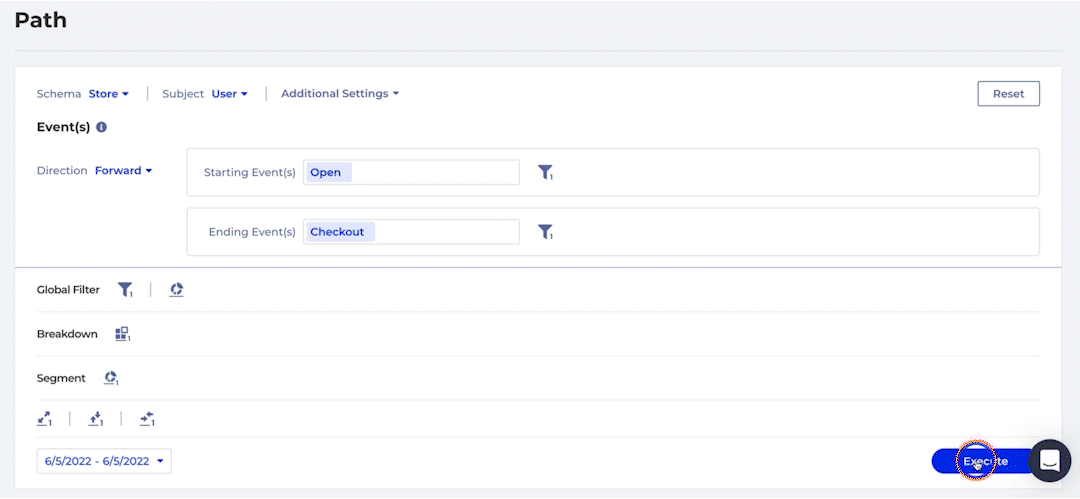
Key Features:
Time Series Analysis
Visualize metrics over time using Kubit’s Query report. You can break down metrics by valuable data points to quickly surface high and low performance. Drill deeper into each data point for granular information. Data can be visualized using line bars, stacked bars, or pie charts.
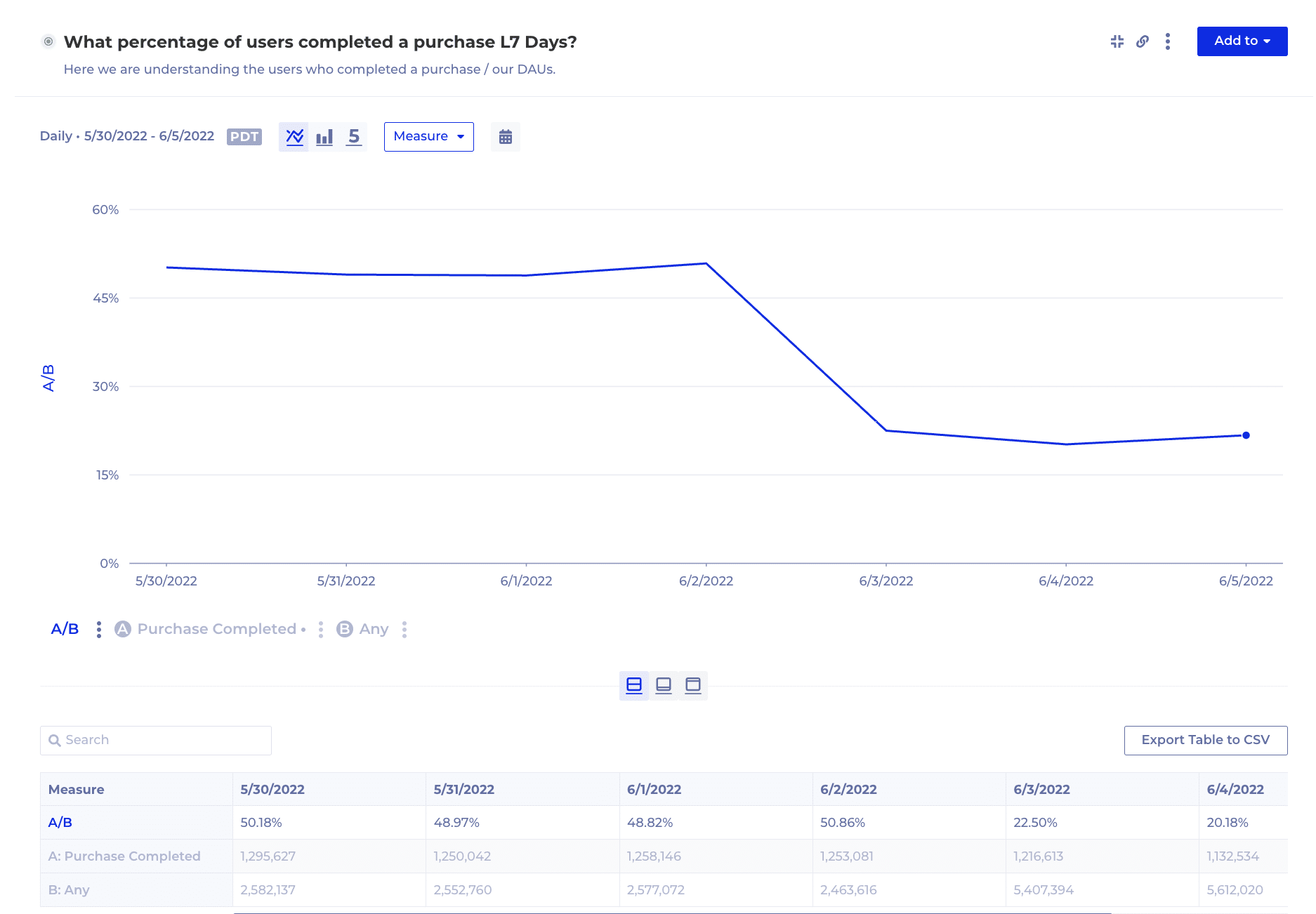
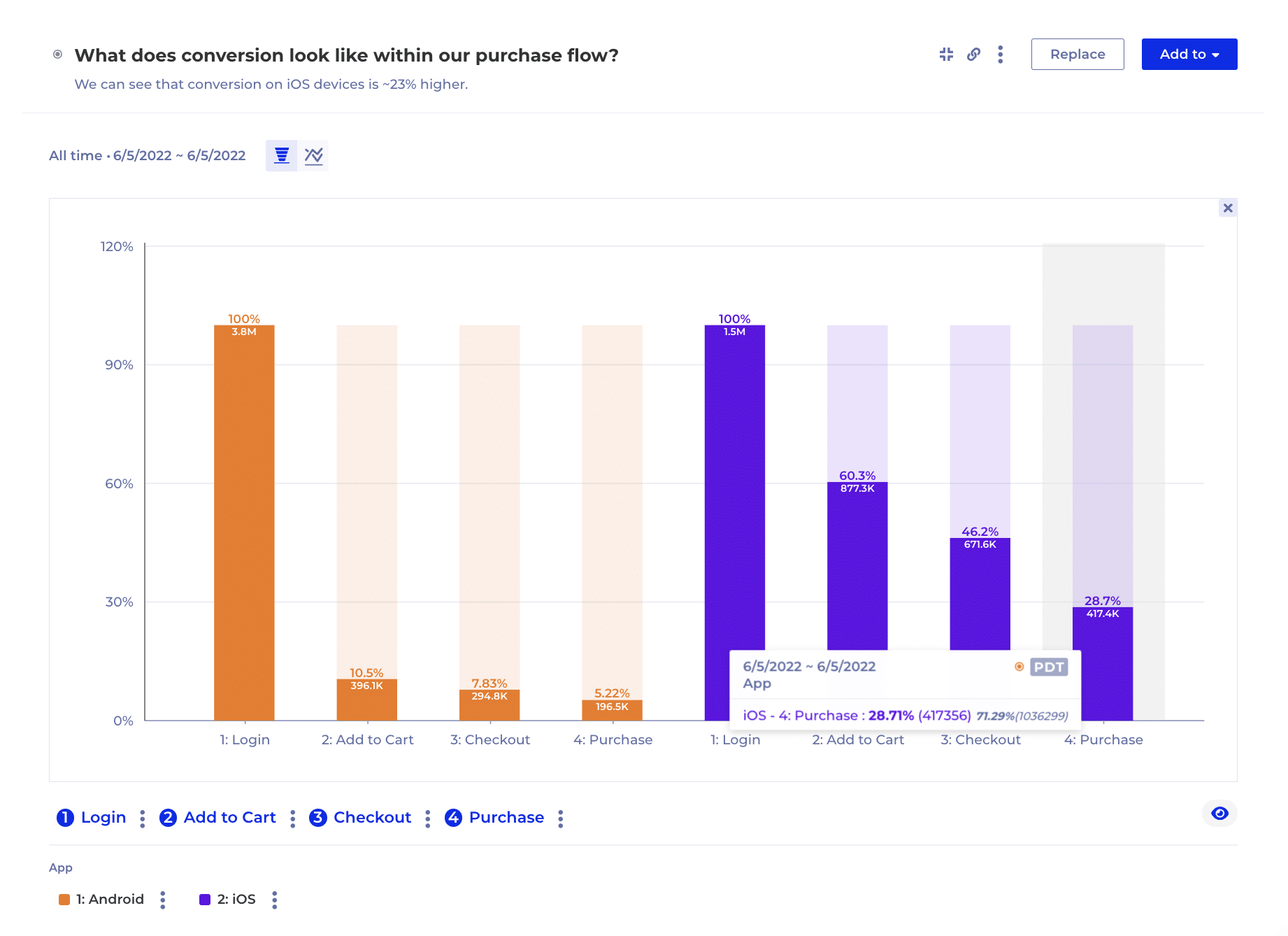
Funnel Conversion
Understand how customers are converting through key flows within your product. Within a funnel, you can see drop-off and conversion metrics with the ability to break down or segment funnels based on additional data. Data can be visualized using traditional conversion, conversion over time, and frequency before conversion.
User Retention
Learn which users return, or never come back to utilize core features and how consistently they retain in the following days. You can construct a retention model that fits your needs as well as decide how you want to visualize this critical metric whether it be retention or churn rates. You are able to analyze your Retention Model as a standard retention curve, retention over time, or usage intervals.
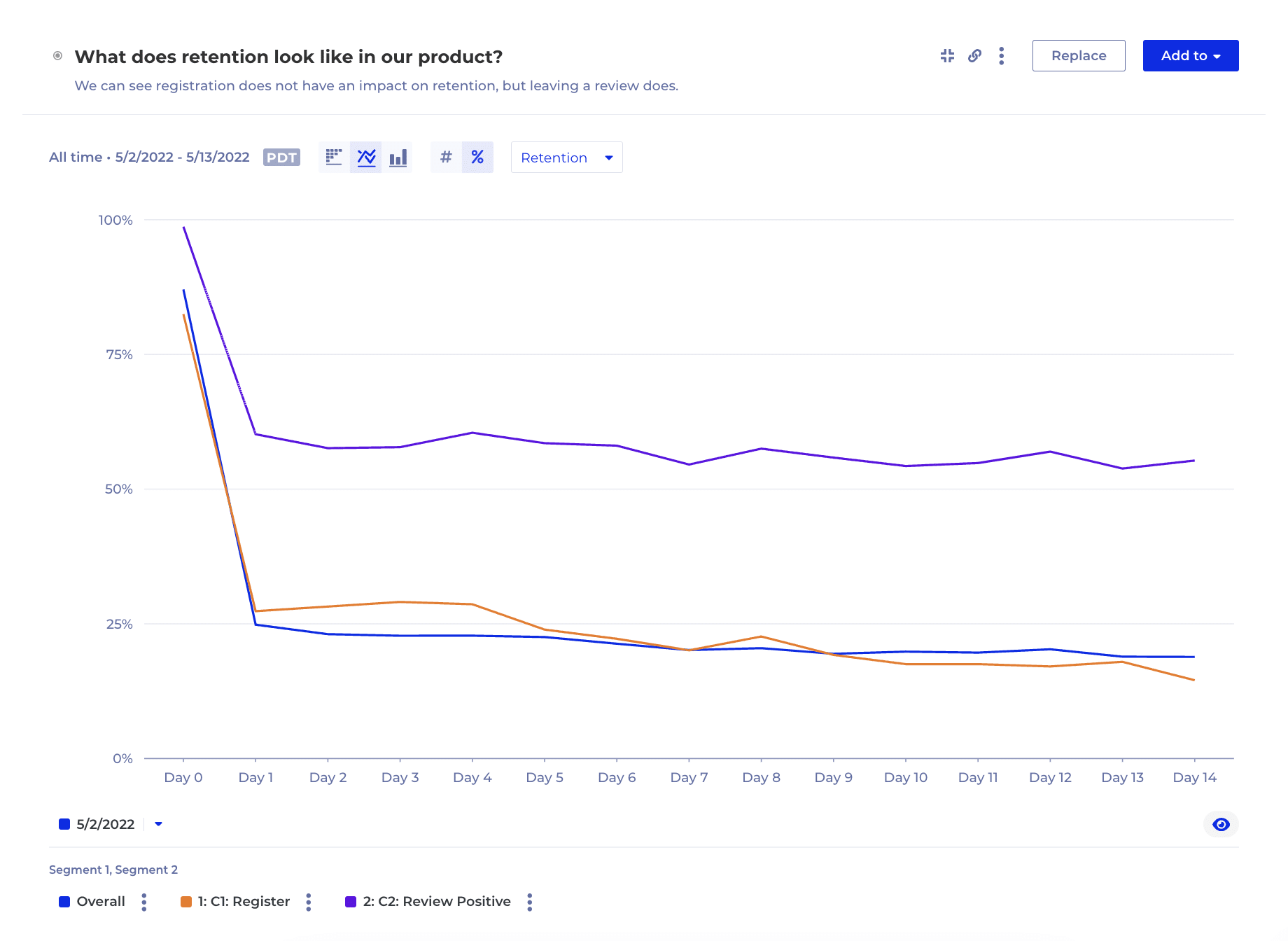
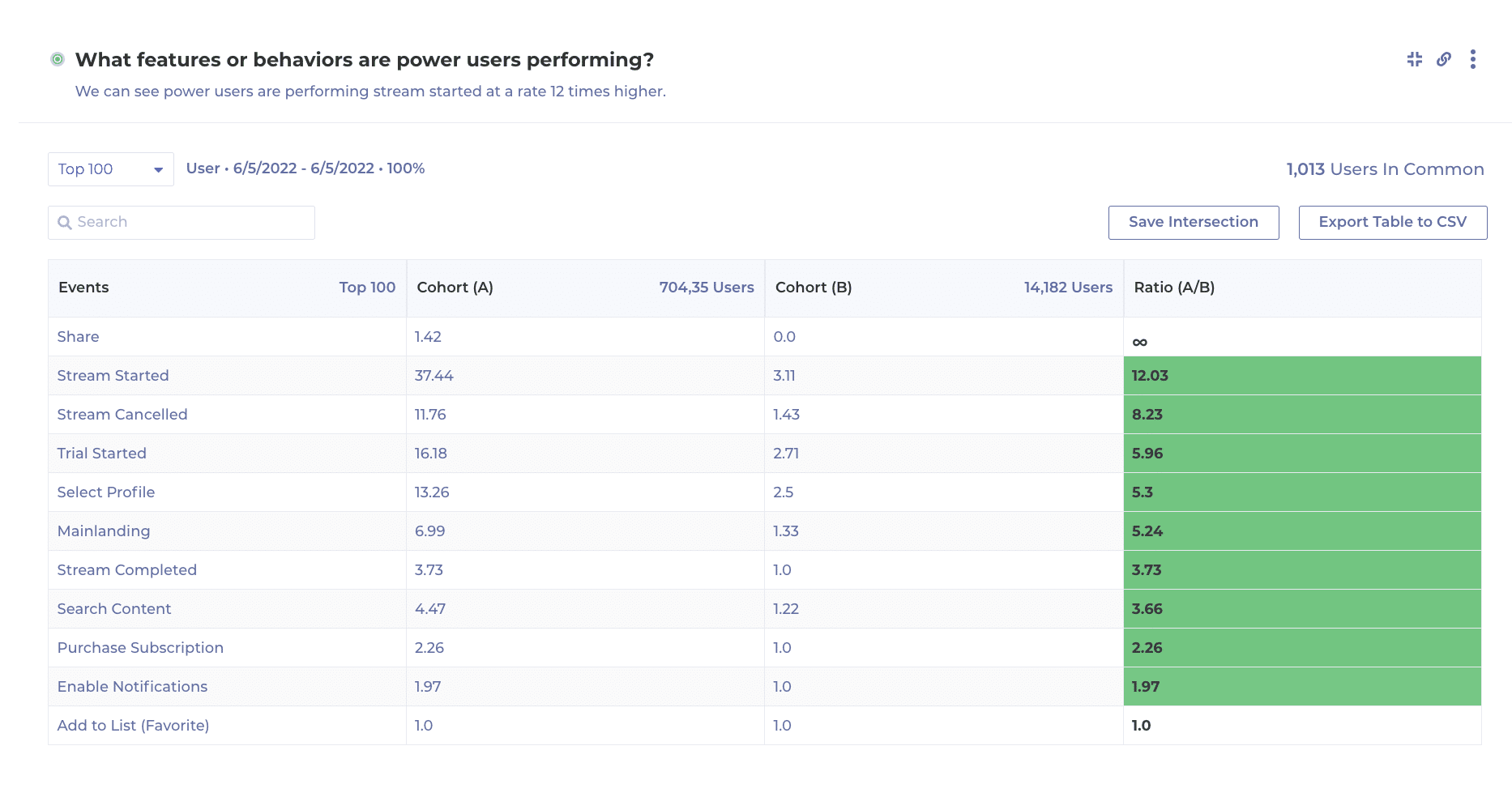
Behavioral Cohorts
Further analyze individuals contained within your data points. You can create cohorts on the fly or by defining them in the Cohort builder. Once a cohort is created, you can reuse it as a segment in any analysis, compare two cohorts, or sync them to a third-party tool to drive targeted outreach.
Kubit Users:
Data Professionals
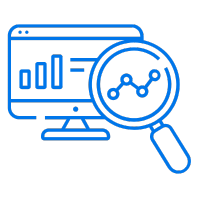
Gain full product/CX insights while keeping your data warehouse the single source of truth.
Product Optimizers
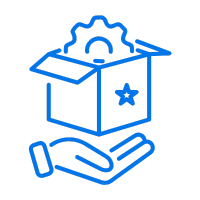
Analyze how users engage with product features to optimize performance and adoption.
Marketers
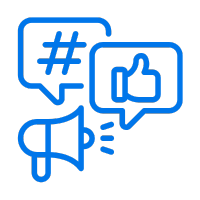
Monitor marketing performance to identify the most effective acquisition channels and content.
Case Studies:
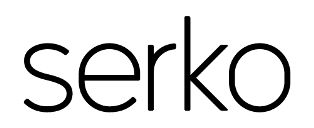
Explore how Serko increased data visualizations 10x by leveraging self-service analytics.
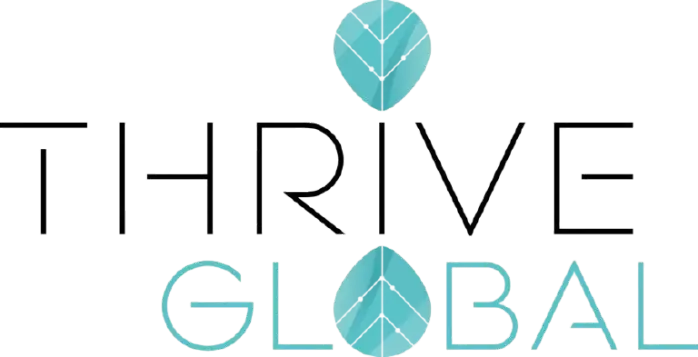
Explore how Thrive accelerated time-to-insights by 95% with a flexible, agile analytics solution.
“Kubit has reduced the workload of my team. It’s so nice to have a truly self-service tool, one where we can teach the Product Managers ‘how to fish,’ so they can then work on their own—whether that’s monitoring certain activities by themselves, or digging into specific user actions that might be really important to them.”
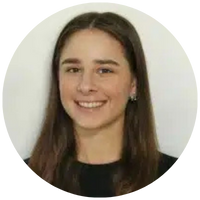
Isabella Poleo
Senior Product Analyst
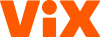
“With Kubit, if I have a question pop into my mind about user behavior, within a minute or two, I can get an answer.”
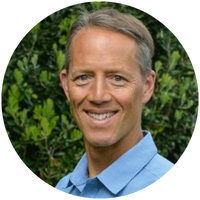
Randall Britten
Senior Data Scientist
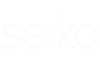
“Within a month of onboarding Kubit we were able to analyze and improve our onboarding flows, increasing ROI by over 30%, which paid for the whole year of service many times over.”
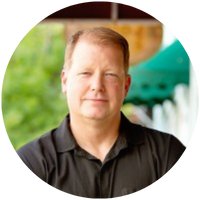
Daniel Todd
CEO
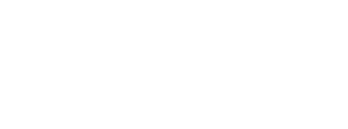
“Kubit has reduced the workload of my team. It’s so nice to have a truly self-service tool, one where we can teach the Product Managers ‘how to fish,’ so they can then work on their own—whether that’s monitoring certain activities by themselves, or digging into specific user actions that might be really important to them.”
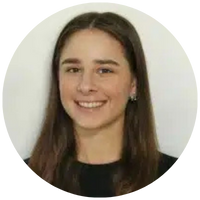
Isabella Poleo
Senior Product Analyst
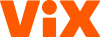
“With Kubit, if I have a question pop into my mind about user behavior, within a minute or two, I can get an answer.”
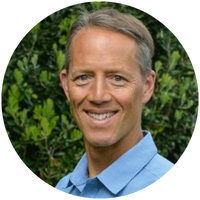
Randall Britten
Senior Data Scientist
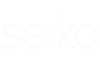
“Within a month of onboarding Kubit we were able to analyze and improve our onboarding flows, increasing ROI by over 30%, which paid for the whole year of service many times over.”
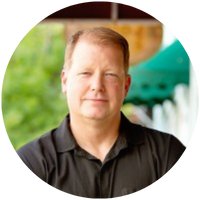
Daniel Todd
CEO
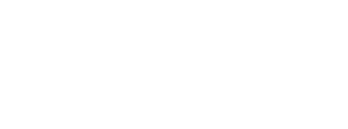
“Kubit has reduced the workload of my team. It’s so nice to have a truly self-service tool, one where we can teach the Product Managers ‘how to fish,’ so they can then work on their own—whether that’s monitoring certain activities by themselves, or digging into specific user actions that might be really important to them.”
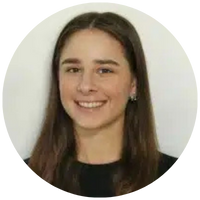
Isabella Poleo
Senior Product Analyst
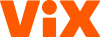
See Kubit in Action:
View an interactive demo of Kubit to explore how you can transform your warehouse data into actionable insights.
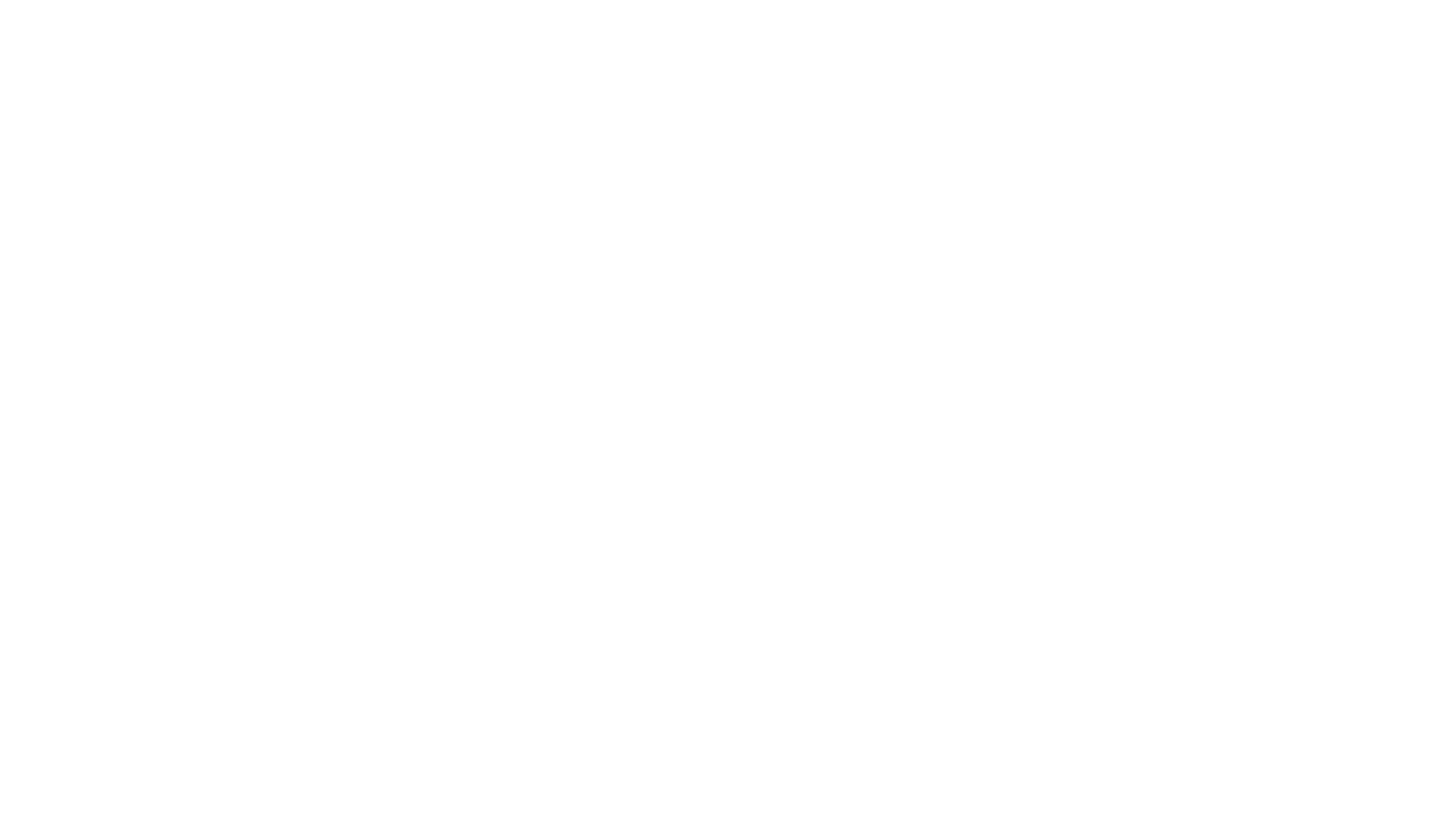
View the Demo:
Explore Our Latest Blogs:
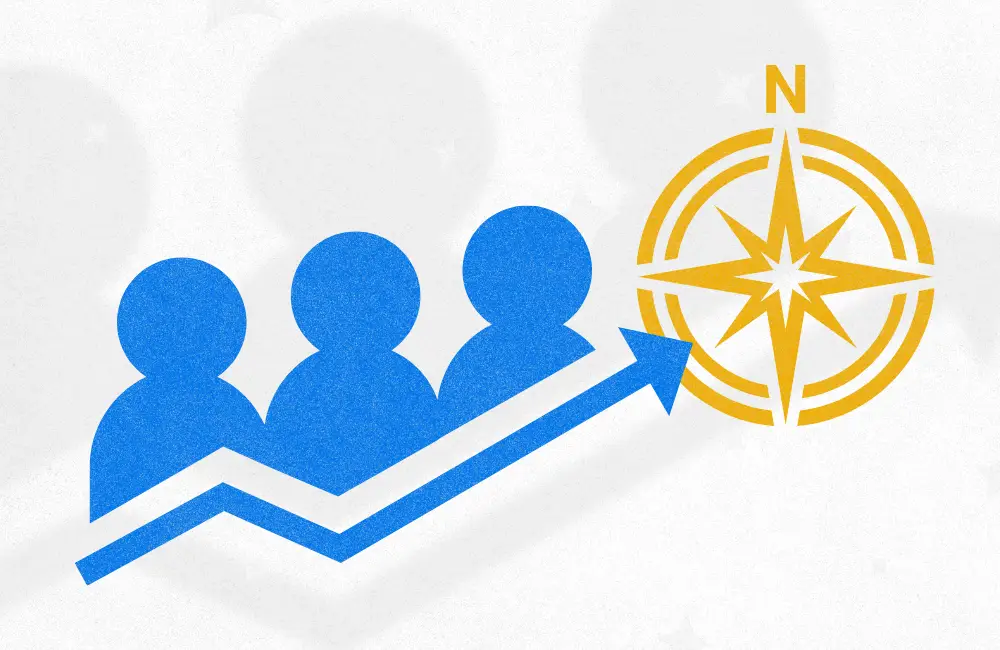
The North Star Metric has become a staple in product strategy. It promises clarity, alignment, and a unified focus ac...
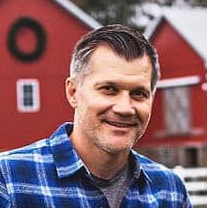
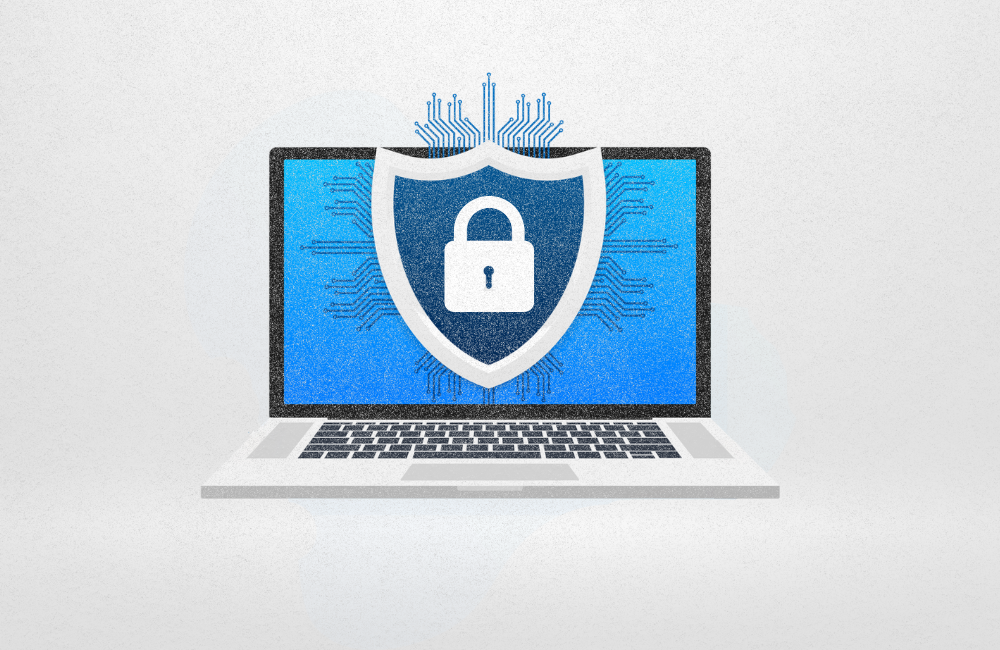
As businesses increasingly rely on data for decision-making, maintaining control over that data has become a critical...
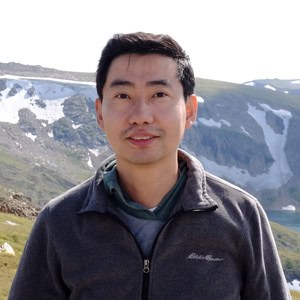
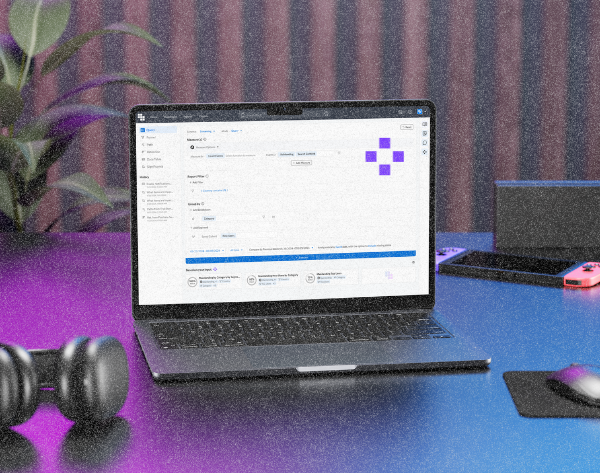
LOS ALTOS, March 18, 2025 – Kubit, a leading customer journey analytics platform, has announced the launch of Kubit L...
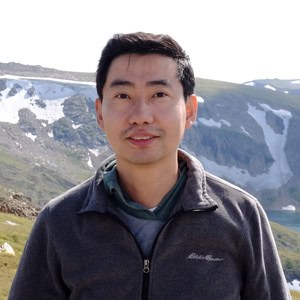
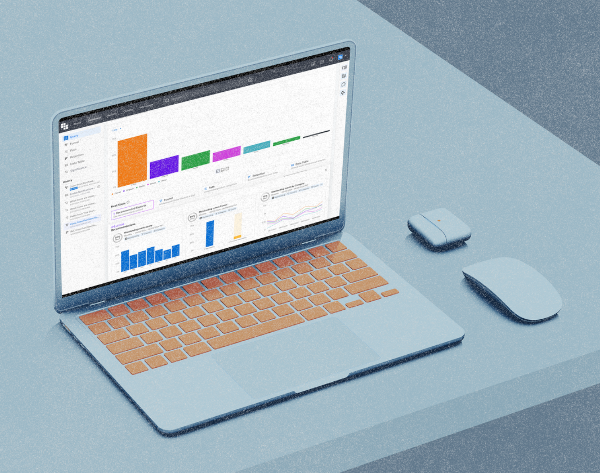
The role of AI data analytics in business intelligence is rapidly expanding, yet many organizations still struggle to...
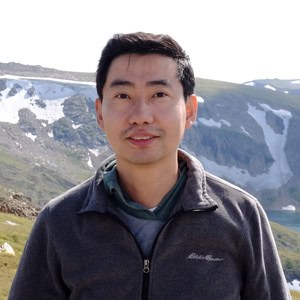