Merriam-Webster defines an anomaly as “something different, abnormal, or not easily classified.” In data science, anomalies usually create a need to research more comprehensive contextual databases to understand the root cause–in other words, a lot of time.
Not all anomalies are unsolved. Several science-based disciplines have successfully applied qualitative research to explain these deviations, and much of the time, the root causes are due to (seemingly) unrelated influences. And, different anomalies can happen in the same space–like the heart. A new generation of babies with heart problems in Russia wasn’t due to people with a particular DNA having more children; it was because of Chernobyl. Alternatively, Ebstein’s anomaly is a disease that causes malformation of the heart, and the root cause is unknown. In nature, anomalies are everywhere, from sea surface temperatures to global magnetic anomalies caused by rock composition–specifically, minerals that create various levels of magnetism.
To figure that out, though, think of all the series of statistics they had to painstakingly enter into a Microsoft Excel document before more advanced programs came about! Even with today’s data-savvy technology, there are plenty of anomalies (especially in space) that we can’t (yet) explain.
The good news is that anomalies are much easier to solve in contexts like product marketing or development, thanks to evolving technology like smarter software that facilitates access to better data. Most anomalies are imaginary, which is why it’s so important to distinguish them quickly from real market events that require action. Let’s say a video game company sees a sudden uptick in the number and rate at which people are “beating” a game. The gaming company can use data mining to “identify the data samples that do not conform to an expected behavior” to explain the anomaly. (Data shows that 40% of gamers admitted to cheating in video games by using a bot.)
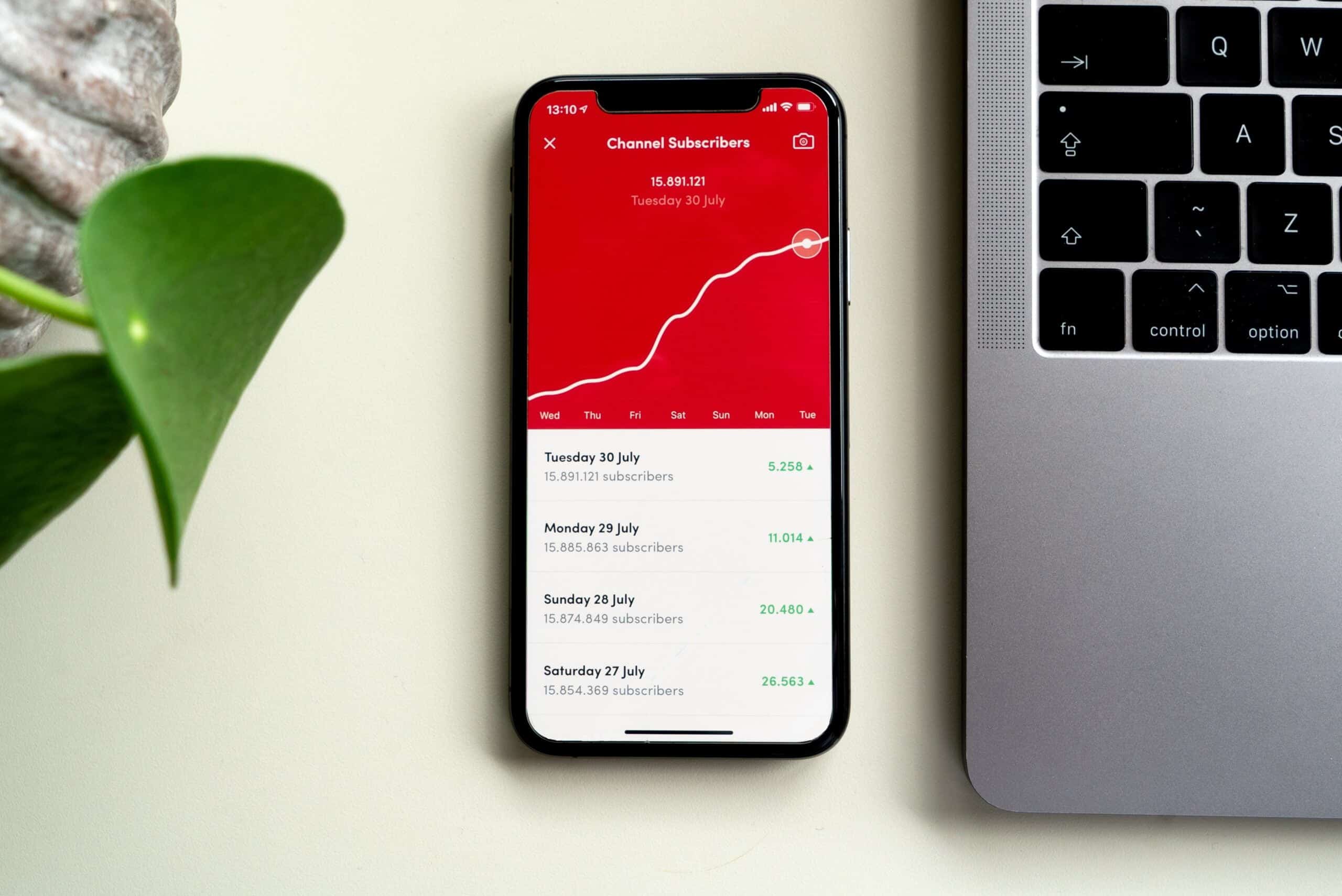
Data-driven businesses rely on algorithms to assess what customers want, as well as predict where their interests are developing. To do this successfully, they need to go beyond pulling information into their system and leverage advanced analytics to see the bigger picture (statistical classification); here’s how the FDA taps into various data sources to understand anomalies. This type of deep learning has made modern-day anomaly detection–and remediation–more accessible to businesses. But for companies that aren’t tapping into crucial problem-solving tools like historical data sets, a “data outlier” can be a frustrating and expensive dead-end. Even with software that provides prediction and anomaly detection, the burden remains on the end-user to answer “why?”
At Kubit, we knew this was a severe pain point–thanks to our collective decades of experience in data analysis–which is why our Augmented Analytics feature uses Artificial Intelligence and Machine Learning to identify immediately the root cause of anomalies. How effective is it to have tech flag an anomaly, but not help users take the steps to resolve it? We believe Kubit closes that gap.
To learn more about anomalies in a business context, read this article that describes top known anomalies such as the “January Effect”–some may be familiar to your company.